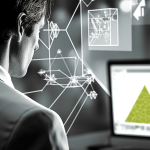
ESG platforms: a solution, not a problem
February 10, 2024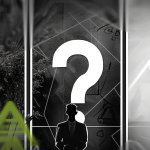
What to consider when choosing an ESG reporting solution
March 31, 2024
AI and ESG: the future of data analysis
In the context of ESG management tools, there is a wide range of functionalities that everyone pays attention to:
- Data Analytics and Insights: The ability to process and analyze large datasets to generate useful information.
- Artificial Intelligence: Supporting data analysis, scenario prediction (“what if” analysis), and other advanced functionalities.
- Risk Management: Identifying, assessing, and managing risks related to ESG factors.
- Goal Management: Setting and monitoring progress towards achieving established ESG goals.
However, for these functions to be effectively realized, it is crucial to have the appropriate amount, quality, and completeness of historical data. The challenge, however, is that ESG data is dispersed both within the organization and outside of it, such as across the entire value chain. There is a misconception that data collection is limited to reporting at the highest organizational level, which is far from the truth. The reality shows that without the right tool, it is difficult to gather historical data at the appropriate level of granularity.
Key elements of reliable ESG report data
These data are crucial not only for calculating ESG indicators but also for identifying areas where interventions can bring significant benefits. This underscores the need to collect data at all organizational levels to fully understand the company’s impact on sustainability and manage this information effectively.
To achieve this, it is necessary to:
- Collect all necessary qualitative and quantitative data from across the organization at the lowest possible level.
- For quantitative data, gather data in absolute terms (e.g., m³), not in averages or percentages, to enable proper data aggregation.
- Ensure all necessary data are collected in appropriate units of measurement, along with source documentation.
- Ensure completeness and currency of data from different business systems.
- Collect data from users, both internal and external, ensuring it is not anonymous – accountability for data submission impacts quality.
- Aggregate data collected “from the bottom” of the organization at higher levels through an acceptance workflow.
- Collect historical data with varying time resolutions, not just annual, but also monthly or quarterly, to allow analysis of changes over time.
By adopting this approach, we open the possibility of building a detailed history and institutional memory, enabling in-depth analysis for setting realistic goals, managing risk, etc. We can then be confident that our data is high quality, verified, and confirmed by appropriate data owners. With such data and a transparent path of its collection, including who is responsible for specific data, we can also relatively easily pass audits of such data.